Director
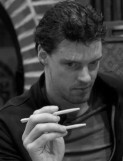
Geoff Nitschke (PhD VU Amsterdam)
Email : gnitschke@cs.uct.ac.za
Research interests : Artificial Life, Evolutionary Machine Learning, Neuro-Evolution, Evolutionary Robotics, Swarm Intelligence and Robotics.
Research profile : Geoff Nitschke is head of the Evolutionary Machine Learning group and Associate Professor at the Department of Computer Science, School of Information Technology, University of Cape Town. He has been working in research on biologically inspired computational intelligence for over 15 years with over 100 publications in peer-reviewed journals, conference proceedings and edited volumes. This research has been supported by grants totalling approximately 20 million ZAR from national and international funding agencies. Geoff is a strong proponent of evolutionary computation and robotics, consistently presenting at key conferences such as Artificial Life (ALIFE), Genetic and Evolutionary Computation Conference (GECCO), the IEEE Congress on Evolutionary Computation (IEEE CEC) and the IEEE Symposium series on Computational Intelligence (IEEE SSCI). Geoff is also associate editor of the IEEE Transactions on Artificial Intelligence, Adaptive Behavior and Frontiers in Robotics and AI journals, and participates in numerous international research visits. Post-doctoral research includes a National Research Foundation (NRF) funded position at the Computational Intelligence Research Group, University of Pretoria, South Africa (2009-2011) and a Japan Society for the Promotion of Science (JSPS) funded position at Ikegami Lab, University of Tokyo, Japan (2011-2012). Sabbatical and research visits include invitations to the Computational Intelligence Group, VU Amsterdam, Netherlands (2017), Earth Life Sciences Institute, Tokyo, Japan (2017-2018), Robotics and Autonomous Systems Group, CSIRO, Brisbane, Australia (2019-2020), School of Computing, Edinburgh Napier University, Edinburgh, United Kingdom (2022, 2024), Department of Information Sciences, Ochanomizu University, Tokyo, Japan (2023), the Okinawa Institute of Science and Technology, Okinawa, Japan (2024), Southern University of Science and Technology (SUSTech), Shenzhen, China (2025) and the Centre for the Advanced Study of Collective Behaviour, University of Konstanz, Konstanz, Germany (2025).
Postdoctoral
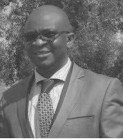
Sabre Didi (PhD UCT)
Email : DDXSAB001@myuct.ac.za
Research topic : Evolving Malware Classifiers
Abstract : Malware attacks remain a critical cyber-security concern, necessitating robust solutions for both individual users and organisations. Deep learning methods have become pervasive tools for malware detection and classification. However, the evolution of malware into sophisticated forms that aim to elude detection poses a formidable challenge to traditional deep-learning methods. Existing techniques for generating adversarial samples often rely on manual feature extraction and white-box models, introducing a gap between the generated samples and real-world scenarios. In response to these challenges, we propose an innovative approach leveraging evolutionary learning for the generation of adversarial samples. This research takes the approach of evolving malware classifiers adapted with evolving adversarial malware samples. Specifically, to demonstrate the efficacy of competitive co-evolution (malware detection versus malware evasion), for the automated adaptation of malware classifiers, where the task performance (malware detection accuracy) of such co-evolved classifiers is compared to established (deep-learning) classifiers given continually adapting malware samples.
Doctoral
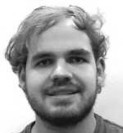
Bingle Kruger (B.Eng Stellenbosch, MPhil UCT)
Email : KRGBIN001@myuct.ac.za
Thesis topic : Automated Strategic Defence of Computer Networks
Abstract : Globalisation has brought increasing dependency on large interconnected networks for the resource (data) control, such as the Internet, communications networks, and power grids. However, such inter-connectivity, coupled with the lack of global view of what is happening in these networks, can lead to tremendous problems in network reliability. For example, small local failures easily propagates to entire networks, causing loss of service and data corruption. Also, deliberate attacks, such as denial of service attacks, viruses and other malware, can easily cause widespread havoc. This research topic proposes investigating the efficacy of automated software agents (self-adapting computer network-based programs) that effectively traverse networks to protect as many resources as possible from failure and malicious attacks. The goal of such automated agents is to maximally restrict the number of resources damaged (due, for example, to intentional malicious attacks or unintentional network hub failures). To address this, we will create a ”resource protection” agent-based system that capture the essential aspects of this problem. That is, a realistic and sophisticated computer network simulation, where defender agents are tasked with protecting network resources before they are damaged by adversarial agents (for example, viruses, malware or denial of service attacks).
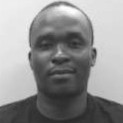
Innocent Sibanda (BTech Hons HIT, MSc Hertfordshire)
Email : SBNINN003@myuct.ac.za
Thesis topic : Evolutionary Dynamics of Asexual Populations at High Mutation Rates
Abstract : Evolutionary dynamics of asexual populations at high mutation rates have long been a central topic of interest to biologists and evolutionary theorists. Biological functions can be robust to changes in sequence; therefore, many different sequences can perform the same function, and when connected with point mutations, these sequences can form a neutral network that allows organisms to explore sequence space without losing function. Neutral network concept is critical in evolutionary biology because it represents a source of genetic variation that can accumulate and spread through a population without significantly impacting fitness. This research explores how neutral network’s topology influences population distribution and mutational robustness in asexual populations at high mutation rates; in addition, this work will investigate possible trade-offs between population distribution and robustness influenced by neutral network topology and, lastly, the effects of interactions between higher-order epistasis and neutral network topology on population distribution and robustness. Datasets with a good fitness landscape will be sourced from the National Institutes of Health database. Combination of artificially generated network science models and data driven experimental approach will be used to carry out this research.
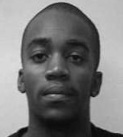
Thabo Ntsoko (MSc.Eng UCT)
Email : NTSTHA019@myuct.ac.za
Thesis topic : Evolutionary Collective Behaviour Transfer
Abstract : This thesis investigates various evolutionary search methods coupled with collective behaviour (multi-agent policy) transfer as a means to more effectively and efficiently solve disparate ranges of collective behaviour (multi-agent) tasks across related problem domains. Specifically, the research investigates the interaction of various behaviour (controller) representations, behaviour evolution and policy transfers methods, on facilitating the effective transfer of evolving robotic controller across various collective robotic tasks -- for example, collective behaviours evolved for a robot group to solve a cooperative reconnaissance task could be transferred (with further online adaptation) to another robot group tasked with cooperative object gathering and transport, and eventually robot behaviour adapted in this task would be transferable to solve more complex collective behaviour tasks.
Master's
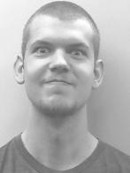
Reece van der Bank (BSc Hons UCT)
Email : VBNREE001@myuct.ac.za
Thesis topic : Evolution of Damage Recovery in Robotic Swarms
Abstract : Swarm robotics is a rapidly expanding field; however, designing control software for robot swarms remains challenging. Existing methodologies often struggle to dynamically adjust to sudden environmental changes or damages within the swarm, while maintaining a similar level of performance. This research proposes extending Multi-dimensional Archive of Phenotypic Elites (MAP-Elites) to evolve robust controllers capable of rapidly adapting swarms to changes across various task environment (e.g., new terrain types), and damage within the swarm, while maintaining a high level of efficiency without further evolutionary adaptation. MAP-Elites evolved controllers will undergo extensive bench marking to assess their adaptability and performance in response to sudden new tasks, environments and damage within the swarm. Evaluations will compare the efficiency (i.e. how well swarm task-performance is maintained) and speed of adaptation (i.e. how quickly the swarm can complete the task, or get back on track) of evolved controllers to existing benchmarks for undamaged swarms.
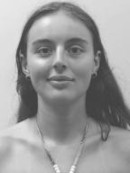
Sian Heesom-Green (BSc Hons UCT)
Email : HSMSIA001@myuct.ac.za
Thesis topic : Neural Complexity Evolution in Changing Environments
Abstract : A supposition is that larger brains evolved to facilitate behavioural adjustments for better survival under changing conditions, where the organisation of the brain also significantly influences cognitive ability and adaptive responses. Previous studies in Evolutionary Robotics (ER) have highlighted that, given a fixed brain size, neural structure complexity increases over evolution, particularly in environments that are context-sensitive with high memory demands. However, the impact of changing environments on the evolution of neural structures alongside brain size remains understudied. This research investigates the evolution of neural complexity, in terms of both brain size and structure, within periodically changing environments. Investigating this may reveal the preferred mechanisms by which organisms evolve to adapt to change. Additionally, the study explores the impact of energy costs associated with brain size and its effect on the evolved robots' task performance and neural complexity, addressing whether larger brains (neural networks) are always beneficial or if specific neural wiring is more important.
Alumni
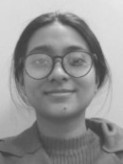
Farzana Haque Toma (BSc Hons BracU, MSc UCT)
Email : TMXFAR001@myuct.ac.za
MSc Thesis : Design Problem Optimization with Multi-Objective Evolutionary Algorithms. [PDF].
Current : Lecturer, Computer Science Department, University of Cape Town, South Africa.
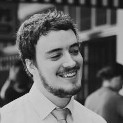
Jeremy Breytenbach (B.Eng Pretoria, MSc UCT)
Email : BRYJER002@myuct.ac.za
MSc Thesis : Exploring the Impact of Novelty and Objective-directed Evolution in Company with MAP-Elites and HyperNEAT. [PDF].
Current : Head of Sales and Marketing, Opti-Num Solutions, Johannesburg, South Africa.
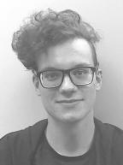
Bilal Aslan (BSc Hons UCT, MSc UCT)
Email : ASLBIL001@myuct.ac.za
MSc Thesis : Multi-Objective Evolutionary Algorithms For Product Design.[PDF].
Current : Research Assistant, Computer Science Department, University of Cape Town, South Africa.
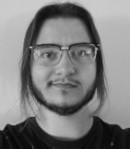
Brandon Gower-Winter (BSc Hons UCT, MSc UCT)
Email : GWRBRA001@myuct.ac.za
MSc Thesis : Self-Adapting Simulated Artificial Societies.[PDF].
Current : PhD Candidate, Information and Computing Sciences, Utrecht University, Netherlands.
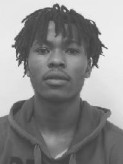
Sindiso Mkhatshwa (B.Eng UCT, MSc UCT)
Email : MKHSIN035@myuct.ac.za
MSc Thesis : Body and Brain Quality-Diversity in Robot Swarms.[PDF].
Current : PhD Candidate, Centre for the Advanced Study of Collective Behaviour, University of Konstanz, Germany.
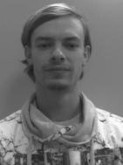
Scott Hallauer (BSc Hons UCT, MSc UCT)
Email : HLLSCO001@myuct.ac.za
MSc Thesis : The Impact of Behavioural Diversity in the Evolution of Multi-Agent Systems Robust to Dynamic Environments.[PDF].
Current : Software Engineer, Amazon Web Services, Cape Town, South Africa.
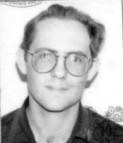
Leon Coetzee (B.Bdg.A Port Elizabeth, MSc UCT)
Email : leon.coetzee@uct.ac.za
MSc Thesis : Evolution of Sun-Shades Outside Building Facades.[PDF].
Current : CIT Liaison Officer, School of Architecture, Planning and Geomatics, UCT, Cape Town, South Africa.
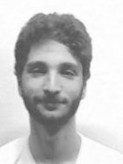
Sasha Abramowitz (BSc Hons UCT, MSc UCT)
Email : ABRSAS002@myuct.ac.za
MSc Thesis : Scalable Hierarchical Evolution Strategies.[PDF].
Current : Software Engineer, InstaDeep Ltd, Cape Town, South Africa.
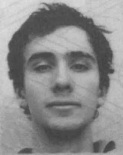
David Jones (BSc Hons UCT, MSc UCT)
Email : JNSDAV026@myuct.ac.za
MSc Thesis : Gaining Perspective with an Evolutionary Cognitive Architecture for Intelligent Agents.[PDF].
Current : Software Engineer, Maholla, Cape Town, South Africa.
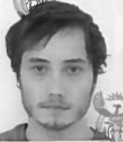
Rob Maccallum (BSc Hons Rhodes, MSc UCT)
Email : MCCROB015@myuct.ac.za
MSc Thesis : Automatic Hit-to-Lead Optimization: Optimizing ligand binding affinity through the application of deep Q-learning to docking simulations.[PDF].
Current : Software Engineer, Zulzi, Cape Town, South Africa.
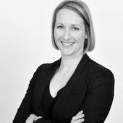
Celia Pienaar (LLM UNISA, LLB Stellenbosch, MIT UCT)
Email : PNRCEL002@myuct.ac.za
MSc Thesis : Machine Learning in Predictive Analytics on Judicial Decision-Making.[PDF].
Current : Legal Services Improvement Manager, Bowmans, Johannesburg, South Africa.
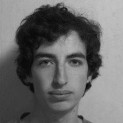
Matthew Cherry (BSc Hons UCT, MSc UCT)
Email : CHRMAT011@myuct.ac.za
MSc Thesis : Distributed Autonomous Intersection Management with Neuro-evolution.[PDF].
Current : Software Engineer, Mystic AI, London, UK.
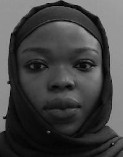
Tassallah Amina Abdullahi (BSc Hons UCT, MSc UCT)
Email : ABDTAS008@myuct.ac.za
MSc Thesis : Predicting Diarrhoea Outbreak with Climate Change.[PDF].
Current : PhD Student in Machine Learning, Brown University, Providence, Rhode Island, USA.
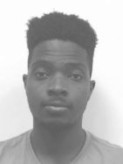
Martin Ombura (BCom Hons UCT, MSc UCT)
Email : OMBMAR001@myuct.ac.za
MSc Thesis : The Performance of Coevolutionary Topologies in Developing Competitive Tree Manipulation Strategies for Symbolic Regression. [PDF].
Current : Software Engineer, GoDaddy, Cape Town, South Africa.
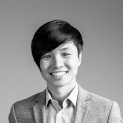
Allen Huang (BSc Hons UCT, MSc UCT)
Email : HNGALL001@myuct.ac.za
MSc Thesis : Neuro-Evolution Search Methodologies for Collective Self-Driving Vehicles. [PDF].
Current : Head Of Business Intelligence, Sameday Health, Los Angeles, California, USA.
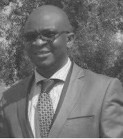
Sabre Didi (MSc NUST Zimbabwe, PhD UCT)
Email : DDXSAB001@myuct.ac.za
PhD Thesis : Neuro-Evolution Behavior Transfer for Collective Behavior Tasks. [PDF].
Current : Postdoctoral Fellow, University of Cape Town, South Africa.
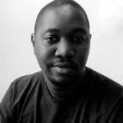
Edmore Moyo (BSc Hons NUST Zimbabwe, MSc UCT)
Email : MYXEDM001@myuct.ac.za
MSc Thesis : Accelerated Cooperative Co-Evolution on Multi-core Architectures. [PDF].
Current : Software Engineer, Allan Gray Proprietary Limited, Cape Town, South Africa.
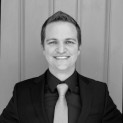
Ryan Goss (MTech NMMU, PhD UCT)
Email : GSSRYA001@myuct.ac.za
PhD Thesis : APIC: A Method for Automated Pattern Identification and Classification. [PDF].
Current : Technical Director, Rubrik, Inc., Palo Alto, USA.
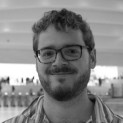
David Shorten (BSc Hons UCT, MSc UCT, PhD USYD)
Email : SHRDAV015@myuct.ac.za
MSc Thesis : Spectral Analysis of Neutral Evolution. [PDF].
Current : Postdoctoral Researcher, School of Mathematical Sciences, Faculty of Engineering, Computer and Mathematical Sciences, University of Adelaide, Australia.
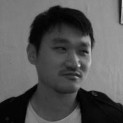
Sunrise Wang (BSc Hons UP, MSc UCT, PhD UGA)
Email : WNGSUN001@myuct.ac.za
MSc Thesis : Evolving Controllable Emergent Crowd Behaviours with Neuro-Evolution. [PDF].
Current : Postdoctoral Researcher, Laboratoire J.-L. Lagrange, L’Observatoire de la Côte d’Azur, Nice, France.